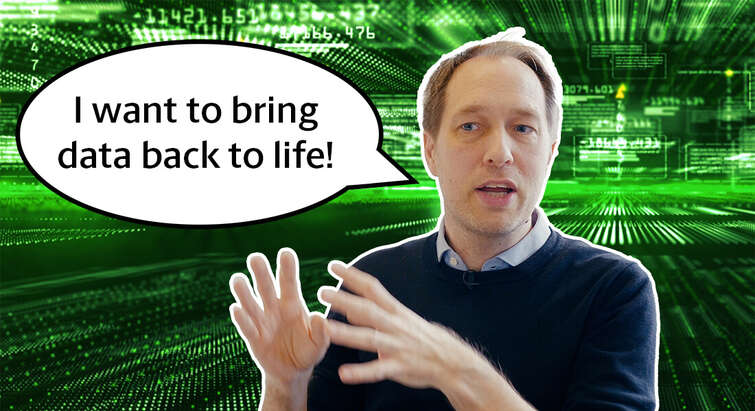
Multi-Modal Bioinformatics in the Rasmussen Group
The Rasmussen Group focuses on the development and application of computational algorithms such as machine and deep learning for analysis and integration of multi-omics and multi-modal data within cardio metabolic disease.
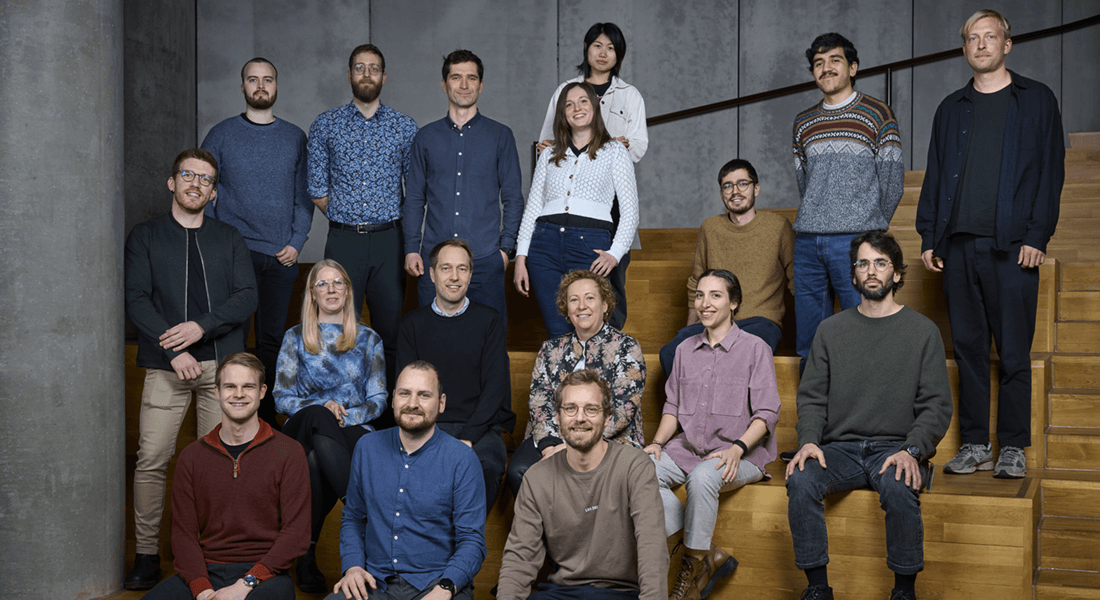
Middle row (from left to right): Leonardo Cobuccio, Terne Torn Jakobsen, Simon Rasmussen, Kirstine Ravn, Foteini Aktypi, Pau Piera Lindez
Bottom row (from left to right): Justus Gräf, Henry Webel, Jonas Meisner
To solve fundamental biological questions related to human health and disease it has become apparent that we must study them from multiple angles using multi-omics and multi-modal data. This is especially important for complex phenotypes such as cardio metabolic diseases.
Associate Professor Simon Rasmussen explains: “Our research aims to develop advanced bioinformatics and computational methods based on recent advances in artificial intelligence. By learning across multiple types of data we are able to better understand diseases and work towards precision health”.
In particular the group focus on analysis of multi-omics data such as genomics, proteomics, microbiome data, clinical records, and electronic health data. By bringing all of this information together we want to translate biological and medical knowledge to the clinic.
“Integration of multi-modal patient data using deep generative models.”
The application of multiple omics technologies in biomedical cohorts has the potential to reveal patient-level disease characteristics and individualized response to treatment. However, the scale and heterogeneous nature of multi-modal data makes integration and inference a non-trivial task. We developed a deep-learning-based framework, multi-omics variational autoencoders (MOVE), to integrate such data and applied it to a cohort of 789 people with newly diagnosed type 2 diabetes with deep multi-omics phenotyping from the DIRECT consortium.
“Deep learning based integration and prediction from whole genome sequences for precisions medicine”.Polygenic risk scores (PRSs) are expected to play a critical role in precision medicine. Currently, PRS predictors are generally based on linear models using summary statistics, and more recently individual-level data. However, these predictors mainly capture additive relationships and are limited in data modalities they can use. We developed a deep learning framework (EIR) for PRS prediction which includes a model, genome-local-net (GLN), specifically designed for large-scale genomics data. The framework supports multi-task learning, automatic integration of other clinical and biochemical data, and model explainability.
“The first deep learning based method for reconstructing microbial genomes from microbiome data”.
Despite recent advances in metagenomic binning, reconstruction of microbial species from metagenomics data remains challenging. Here we develop variational autoencoders for metagenomic binning (VAMB), a program that uses deep variational autoencoders to encode sequence coabundance and k-mer distribution information before clustering. We show that a variational autoencoder is able to integrate these two distinct data types without any previous knowledge of the datasets.
Group Leader
Simon Rasmussen
Professor
Twitter: Simon Rasmussen (@simonrasmu)
LinkedIn: Simon Rasmussen